主讲:董彬, 北京国际数学研究中心
时间:2018年3月21日,星期三,15:00-16:00
地点:物理楼北547
主持/联系:林金泰
摘要:Deep learning continues to dominate machine learning. It is now widely used in many research areas in science and engineering, and has major industrial impacts. In this talk, I will start with a brief review of deep learning and existing work on relating deep neural networks with dynamic systems. I will present my personal understanding of deep learning from the perspective of applied mathematics, which inspired one of our recent work on combining numerical partial differential equations and deep convolutional networks for data-driven modeling of complex dynamics. In this work we designed a transparent deep feed-forward convolutional networks, called the PDE-Net, to accurately predict dynamics of complex systems and to uncover the underlying hidden PDE models. Comparing with existing approaches, which either assume the form of the nonlinear response is mostly known or fix certain finite difference approximations of differential operators, our approach has the most flexibility. Numerical experiments show that the PDE-Net has the potential to uncover the hidden PDE of the observed dynamics, and predict the dynamical behavior for a relatively long time, even in a noisy environment.
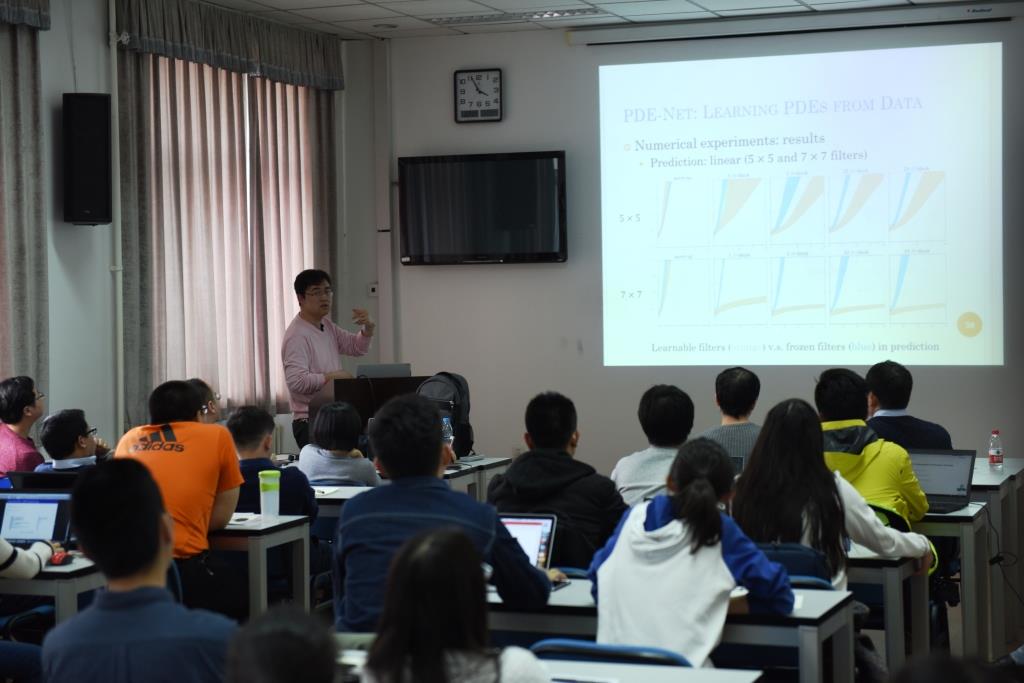